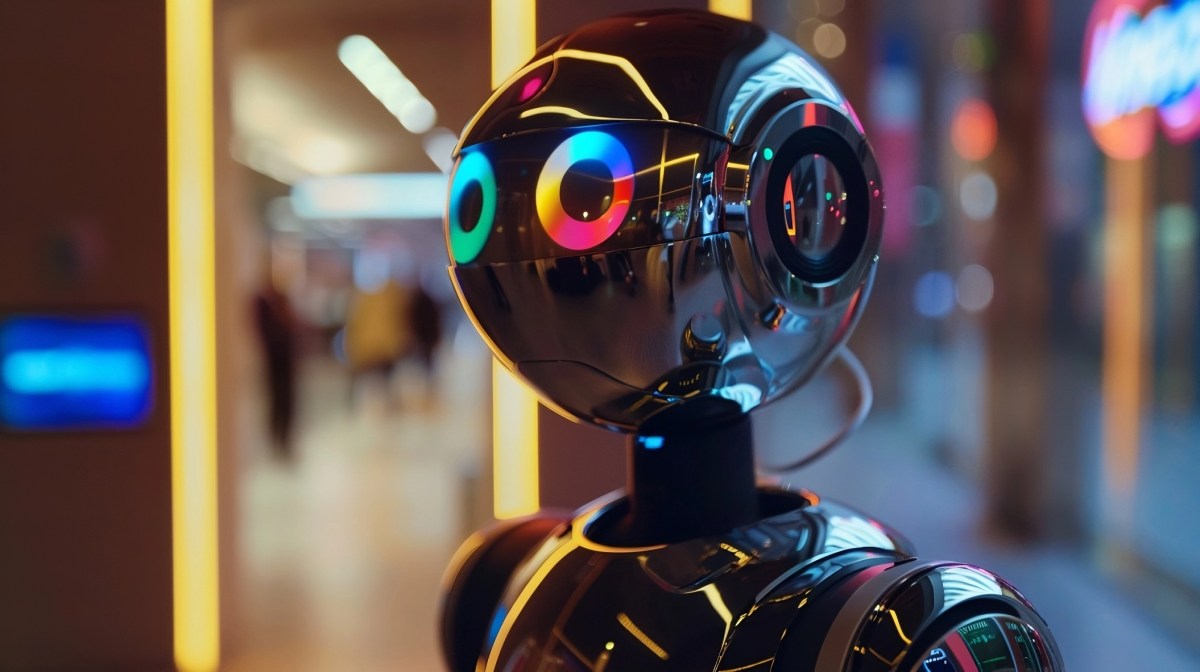
**Table of Contents**
– Introduction
– AI Outperforms Human Fact-Checkers
– The Debate on ‘Superhuman’ Performance
– Cost Savings and Benchmarking Top Models
– Transparency and Human Baselines
– FAQs
**Introduction**
On April 10th, Atlanta will host an event focused on exploring the landscape of security workforce and the use of AI. This article will delve into a recent study by Google’s DeepMind research unit that showcases how an artificial intelligence system can outperform human fact-checkers. We will discuss the implications of this research, the debate surrounding the term ‘superhuman’ performance, the cost savings associated with AI fact-checking, and the importance of transparency in developing such technologies.
**AI Outperforms Human Fact-Checkers**
The study introduced a method called Search-Augmented Factuality Evaluator (SAFE), which utilizes a large language model to evaluate the accuracy of information. SAFE was found to match human ratings 72% of the time and was correct in 76% of cases where there were disagreements between the AI system and human raters. This performance has sparked a debate on what ‘superhuman’ truly means in the context of fact-checking.
**The Debate on ‘Superhuman’ Performance**
AI researcher Gary Marcus raised concerns about the characterization of SAFE’s performance as ‘superhuman’. He argued that benchmarking against expert human fact-checkers is essential to truly demonstrate superhuman capabilities. The specifics of the human raters, including their qualifications and fact-checking process, are crucial for proper context.
**Cost Savings and Benchmarking Top Models**
One of the key advantages of SAFE is cost savings, as using the AI system was significantly cheaper than employing human fact-checkers. The study evaluated the factual accuracy of 13 top language models and found that larger models generally produced fewer factual errors. However, even the best-performing models generated false claims, highlighting the importance of automatic fact-checking tools like SAFE.
**Transparency and Human Baselines**
While the SAFE code and dataset have been open-sourced, more transparency is needed around the human baselines used in the study. Understanding the background and process of human raters is crucial for assessing SAFE’s capabilities accurately. Rigorous benchmarking against human experts, not just crowdworkers, is essential to measure true progress in automated fact-checking.
**FAQs**
1. What is the Search-Augmented Factuality Evaluator (SAFE)?
2. How does SAFE use Google Search results to evaluate the accuracy of information?
3. What are the advantages of using AI for fact-checking compared to human fact-checkers?
4. Why is transparency important in developing automated fact-checking tools?
5. How can benchmarking against human experts improve the accuracy of AI fact-checking systems?